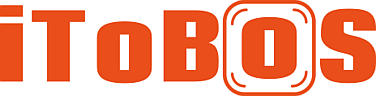
Full name: | Intelligent Total Body Scanner for Early Detection of Melanoma |
Number: | Horizon 2020 - 965221 |
Start date: | 2021. 04. 01. |
End date: | 2025. 03. 31. |
Participants: |
|
Project homepage: | https://itobos.eu/ |
The aim of the iToBoS project is to train an AI system able to integrate information from different sources, assessment of individual moles while considering the specific characteristics of each patient. With systematic successive explorations of a patient, the system will be able to also robustly determine the changes occurring in the individual moles, a key feature held as one of the most informative in the detection of skin cancer. The proposed holistic approach will enable physicians to diagnose skin diseases earlier and with higher accuracy, thus increasing effectiveness and efficiency in personalized clinical decision making.
Current state-of-the-art AI systems for detection of melanomas using a single dermatoscopic image of the patient, fail to “understand” the naevus type of the patient. This naevus type refers to the typical (prevailing) pattern of the patient’s naevi. Clinical practice has proved that only the evaluation of all naevi allows us to define the “naevus type” of the patient examined, and more attention should be directed towards naevi not consistent with the prevailing naevi pattern.
The proposed novel skin scanner will seamlessly acquire complete skin imagery comparable to the quality of dermoscopy, but covering most of the patient’s skin. As our AI cognitive assistant analyses the complete skin of the patient, it will be able to detect all the naevi of the patient, defining the naevus type for that specific patient. This approach enables the recognition of ugly ducklings, i.e., detection of a mole that does not resemble the average normal mole of that specific patient, which is something current AI systems cannot do.
The iToBoS full body scanner
iToBoS will provide high prediction quality by fusing different types of data, including medical records, genomics and temporal evolution of lesions, while using the total body maps to detect ugly ducklings (naevus phenotype) and measuring the imaging phenotype of that specific patient, i.e. assessment of UV damage, size and number of naevi, and location of each lesion, thus achieving a highly personalised diagnosis. However, high prediction quality is not enough to increase effectiveness and efficiency of treatments: the dermatologist requires explanation and insight for a better understanding beyond standard quantitative performance evaluation. The iToBoS team has proposed in the literature several AI explanation methods, concerning state of the art computer vision datasets, but also a few in the medical domain. The project will also develop explainable AI (XAI) methods focused on the generation of system justifications that will sufficiently and understandably explain the reasoning behind its inference to the dermatologist.